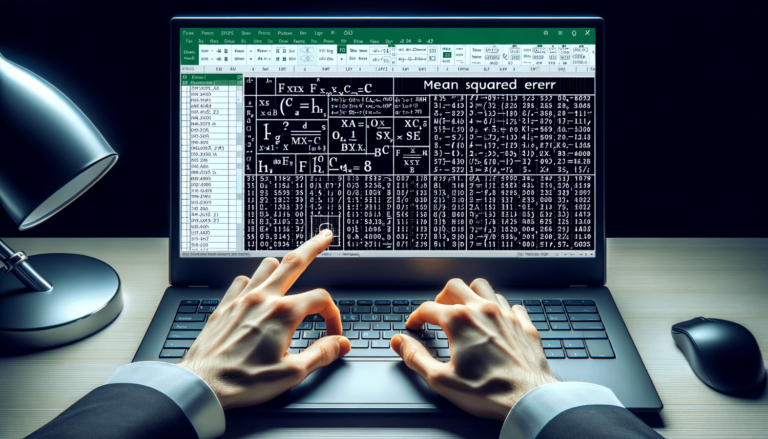
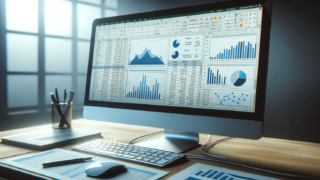
If you’re working with statistical data and want to measure the accuracy of your forecasting model, then Mean Squared Error (MSE) is your go-to metric. Excel provides a straightforward way of calculating MSE without the need for any complex coding or statistical knowledge. By following a few simple steps, you can quickly calculate MSE and evaluate your models’ performance.
Mean Squared Error (MSE) is a commonly used metric to measure the accuracy of forecasting models. It measures the average of the squared differences between predicted and actual values.
MSE offers a more comprehensive measure of the forecasting model’s accuracy than simple metrics like the mean error or mean absolute error. High MSE values indicate that the model’s predictions are consistently off by a larger magnitude.
Assuming you have a set of predicted values and actual values, arrange your data into two columns in Excel, with the actual values in column A and the corresponding predicted values in column B.
To find the difference between the predicted and actual values, you will need to create a new column in Excel, labeled “Difference.” In the first row of this new column, type in the formula “=B1-A1.” Then, drag the formula to the bottom of the column so that it applies to all the rows in your dataset. This will populate the entire column with the difference between the predicted and actual values.
In a new column (label it “Squared Difference”), use the formula “=C1^2” in the first row. Then, apply it to the rest of the column by dragging the formula down. This column will now show the squared difference between each predicted value and its corresponding actual value.
Calculate the average of the squared differences by using the Excel formula “=AVERAGE(D1:Dn)” where n is the number of rows of data you have. This will give you the Mean Squared Error (MSE).
Now that you have your MSE calculation, you can use it to compare the accuracy of different forecasting models. By identifying which models have the lowest MSE, you can determine which models have the best predictive power.
There is no definitive answer to what constitutes a “good” MSE value since it depends on the nature and complexity of the forecasting problem. As a general rule of thumb, an MSE value of less than 1 is a sign of a reasonably accurate forecasting model. Conversely, an MSE value greater than 1 indicates that the model is not a good fit for the data.
While MSE is a useful performance metric, it has some limitations. For instance, it tends to penalize large errors more than small errors, which could skew the results in the presence of outliers or extreme values.
MSE is also sensitive to the scale of the variables in the dataset. Therefore, it may not be the best choice of metric where different variables have different scales or units of measurement.
There are several alternative performance metrics you can use alongside or instead of MSE to evaluate the forecasting model’s accuracy, such as:
The choice of an appropriate performance metric depends on the nature of the data and the specific goals of your analysis.
MSE is a useful metric that can help you measure the accuracy of your forecasting models in Excel. Using Excel’s built-in functions, you can easily calculate MSE and extract insights into your model’s performance.
While MSE has its limitations, you can use it alongside other performance metrics to get a more comprehensive view of your model’s accuracy. With this knowledge, you can refine your forecasts and achieve better insights into your business or research questions.
Here are some common questions about Mean Squared Error (MSE) in Excel:
Root Mean Squared Error (RMSE) is similar to MSE but adds an extra step of taking the square root of the average squared differences. The use of RMSE is beneficial over MSE when you want to measure the accuracy of your forecasting model in the units of the original data.
No, MSE cannot be negative as it is the average of the squared differences between predicted and actual values. Since the squared differences are always positive, the average must also be positive.
If your MSE value is too high, it indicates that your forecasting model is consistently off by a larger magnitude. You may want to re-examine your model assumptions, feature selection, or data cleaning process to make sure it’s accurately capturing the data patterns. Alternatively, you may want to consider other forecasting models and compare their performance metrics.
No, you cannot directly compare the MSE values of two models with different numbers of data points since the MSE is sensitive to the data distribution. A better approach is to use an adjusted form of MSE, such as Mean Absolute Percentage Error (MAPE), which can account for differences in the data size and distribution.
MSE offers a more comprehensive measure of the accuracy of forecasting models compared to other performance metrics such as Mean Absolute Error (MAE) or R-squared. MSE takes into account both the direction and the magnitude of the forecast error, making it a more accurate representation of the model’s predictive power.
Explore the world of Microsoft PowerPoint with LearnPowerpoint.io, where we provide tailored tutorials and valuable tips to transform your presentation skills and clarify PowerPoint for enthusiasts and professionals alike.
Your ultimate guide to mastering Microsoft Word! Dive into our extensive collection of tutorials and tips designed to make Word simple and effective for users of all skill levels.
Boost your brand's online presence with Resultris Content Marketing Subscriptions. Enjoy high-quality, on-demand content marketing services to grow your business.